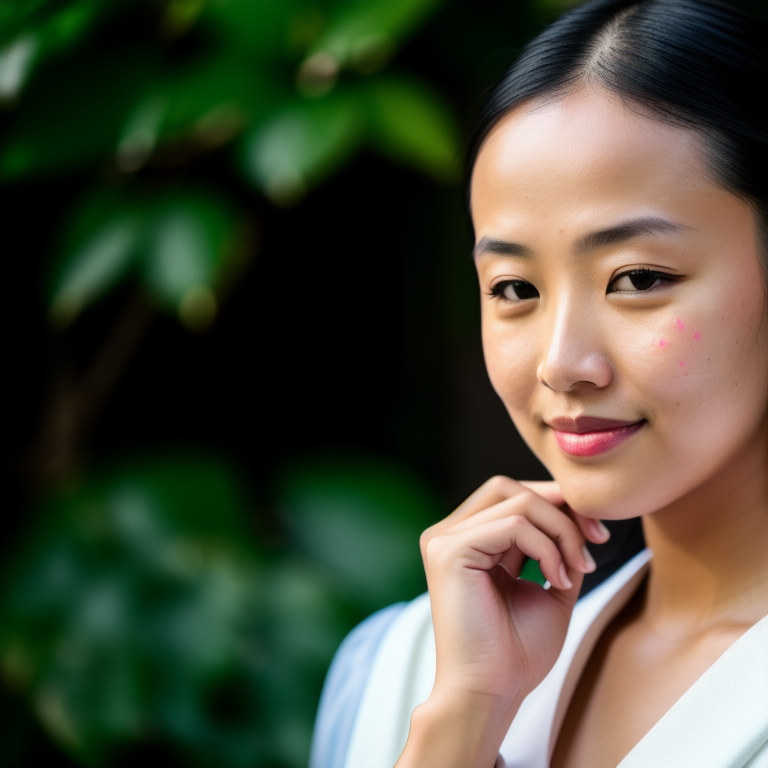
Strategies for Future of Real-time Data Processing with AI Solutions:
Strategies for the Future of Real-Time Data Processing with AI Solutions
In the fast-paced world of technology, the ability to process data in real-time using artificial intelligence (AI) is increasingly crucial for businesses across industries. From finance and healthcare to manufacturing and retail, the integration of AI in real-time data processing is transforming operational capabilities, enhancing decision-making, and providing a competitive edge. This Blog post explores the latest trends, benefits, and practical applications of real-time data processing with AI solutions, along with strategic insights to leverage these technologies effectively.
Understanding Real-Time Data Processing
Real-time data processing involves the continuous input, processing, and output of data, providing immediate insights and enabling instant decision-making. Unlike batch processing, which handles data at scheduled intervals, real-time processing delivers instantaneous data analysis, which is vital for applications requiring immediate action, such as fraud detection in banking or patient monitoring in healthcare.
The Role of AI in Enhancing Real-Time Data Processing
AI enhances real-time data processing by bringing sophisticated analytical capabilities that can learn and adapt over time. Machine learning models, a subset of AI, can analyze large streams of real-time data to identify patterns, predict trends, and make decisions with minimal human intervention.
Latest Trends in AI and Real-Time Data Processing
1. Edge Computing: By processing data near its source, edge computing reduces latency and speeds up response time, which is essential for IoT devices and autonomous vehicles.
2. Automated Machine Learning (AutoML): This technology automates the process of applying machine learning to real-time data, making it more accessible to non-experts and increasing the efficiency of data processing.
3. AI-Driven Predictive Analytics: In sectors like retail and supply chain management, predictive analytics can forecast demand in real-time, optimizing inventory and reducing waste.
4. Natural Language Processing (NLP): Advanced NLP is being used in customer service to process and respond to queries and feedback instantly, improving customer experience.
Benefits of Integrating AI with Real-Time Data Processing
– Enhanced Decision-Making: AI-driven insights help organizations make informed decisions swiftly, which is crucial in environments where conditions change rapidly.
– Increased Efficiency: Automation of data processing reduces the need for manual intervention, speeding up operations and reducing errors.
– Improved Customer Experience: Real-time insights into customer behavior and preferences enable personalized customer interactions.
– Risk Management: Immediate processing and analysis can help in identifying and mitigating risks promptly.
Practical Applications and Real-World Examples
– Financial Services: Banks and financial institutions use real-time data processing to detect fraudulent transactions as they occur. For example, AI algorithms analyze spending patterns and flag transactions that deviate from the norm, preventing potential fraud.
Error fetching quote from OpenAI.
– Healthcare: Real-time data processing enables continuous patient monitoring. AI models can predict patient deterioration before it occurs, allowing for timely interventions. An example is the use of AI in monitoring ICU patients to detect subtle changes in vital signs that might indicate complications.
– Manufacturing: In manufacturing, AI-driven real-time data processing helps in predictive maintenance. Sensors on equipment can detect anomalies and predict failures before they happen, reducing downtime and maintenance costs.
– Transportation: AI enhances real-time data processing in traffic management systems, adjusting signals based on traffic flow to reduce congestion. Autonomous vehicles also rely on real-time data processing to make split-second decisions on the road.
Strategies to Implement AI in Real-Time Data Processing
1. Invest in Quality Data Infrastructure: Robust infrastructure is crucial to handle large volumes of real-time data efficiently.
2. Focus on Scalability: As data volumes grow, the ability to scale processing capabilities without compromising performance is essential.
3. Prioritize Data Security: Implement advanced security protocols to protect sensitive real-time data processed by AI systems.
4. Continuous Learning and Improvement: Regularly update AI models and algorithms to adapt to new patterns and changes in real-time data.
5. Collaborate Across Departments: Ensure that insights generated from real-time data are shared across the organization to enhance overall operational efficiency.
Conclusion
The future of real-time data processing is inextricably linked with advancements in AI technology. By adopting the right strategies and staying abreast of emerging trends, businesses can harness the power of real-time AI-driven data processing to enhance operational efficiency, improve customer satisfaction, and stay ahead in the competitive market. As technology evolves, the integration of AI in real-time data processing will only deepen, unlocking more opportunities and driving innovation across sectors.
Thank you for taking the time to read our blog! We hope you found the information valuable and insightful.
If you have any questions, comments, or topics you’d like us to cover in future posts, please don’t hesitate to reach out.
Stay tuned for more updates, and don’t forget to subscribe to our newsletter for the latest news and insights.
Warm regards,
VisionKraft Consulting